Course description
Join us for an unparalleled learning experience that will transform your understanding of biostatistics and epidemiology.
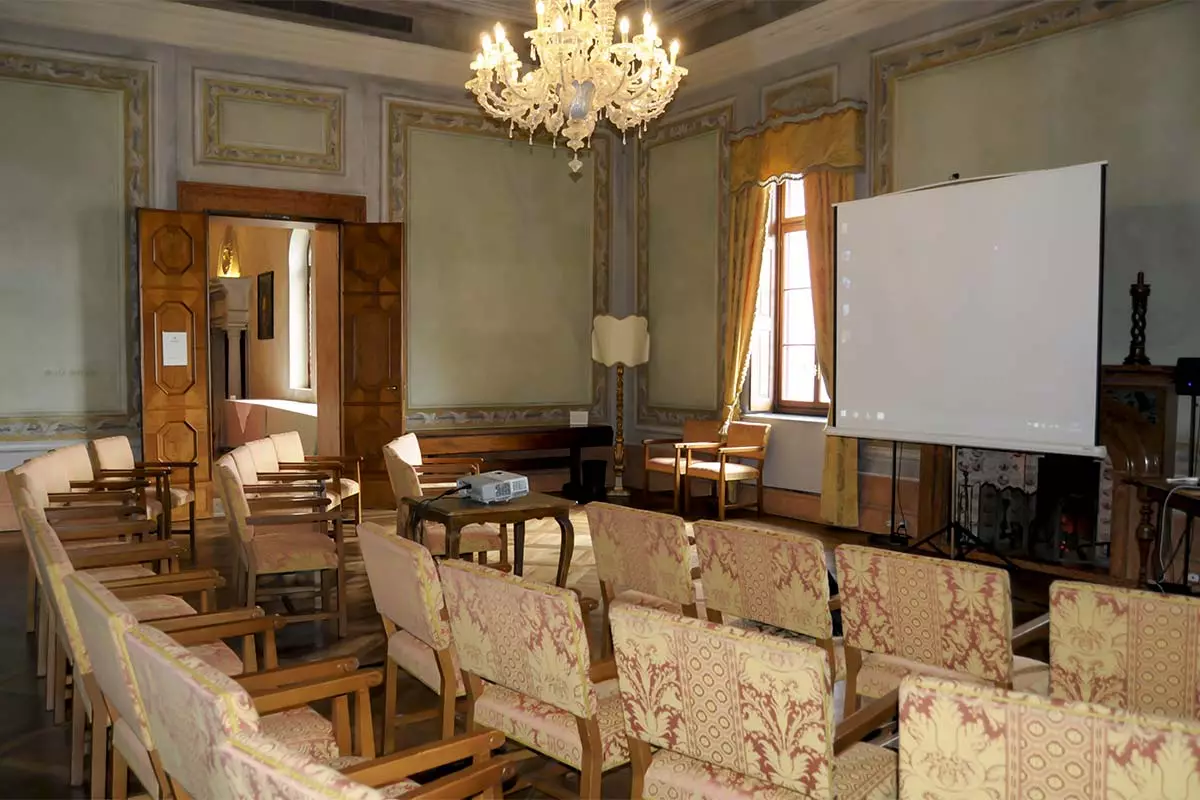
Block 1
Principles of Biostatistics
Nicola Orsini (Karolinska Institutet)
This course introduces you to the fundamental principles and methods of statistics, specifically tailored for applications in the health sciences. You will master descriptive statistics, learn to apply Bayes theorem for updating probabilities, evaluate the accuracy and reliability of diagnostic tests, and understand the differences between population parameters and sample estimates. Additionally, you will develop skills in hypothesis testing, sample size and power calculations, making statistical inferences, and analyzing comparative measures such as mean differences, risk ratios, odds ratios, and rate ratios in both experimental and observational studies. By the end of this course, you will be proficient in fundamental statistical concepts and their application in clinical and epidemiological studies, enabling you to utilize sample data to draw accurate and insightful statistical inferences, and contribute effectively to research and decision-making in the health sciences.
Regression models for continuous outcomes
Matteo Bottai (Karolinska Institutet)
This course is suitable for anyone who wish to learn about linear regression and quantile regression, which are flexible and popular statistical tools to investigate continuous health outcomes and the effect that other factors may have on them. For example, they allow to investigate predictors of outcomes in predictive modes for clinical practice, control for confounders, interactions, modifiers, moderators, and mediators in studying exposures and outcomes in association studies, balance unequal distribution of underlying factors in groups comparisons with observational data, and more. The course introduces these regression methods through simple real-life examples and the use of the Stata statistical software.
Causal Inference in Epidemiology
Michele Santacatterina (NYU Grossman School of Medicine)
In the last few decades, many techniques have been developed to estimate causal effects from observational real-world data. This course will introduce techniques to identify and estimate causal effects from observational real-world data. Specifically, the course will first explain the use of direct acyclic graphs, the potential outcome framework, and identification assumptions to identify causal estimands. The course will then introduce statistical methods to estimate these estimands, such as regression adjustment, inverse probability weighting, matching, and doubly robust estimators. All theoretical concepts will be set into the context of real-life research problems, taken from medicine and epidemiology. Lab sessions in Stata will provide an opportunity for 'hands-on' training in causal inference. Causal inference is an essential research topic in the statistical, medical, epidemiological, and social sciences. By the end of this course, you will be able to identify, estimate and compute causal effects using observational data, thus improving your research and decision-making skills.
Block 2
Principles of Epidemiology
Murray Mittleman (Harvard T.H. Chan School of Public Health)
This course provides an introduction to the skills needed by public health professionals and clinicians to critically interpret the epidemiologic literature. It will provide participants with the basic principles and practical experience needed to develop these skills. This will be accomplished by covering the basic principles and methods of the design, conduct and interpretation of epidemiologic studies, including descriptive studies, observational analytic studies (case-control and cohort), and randomized clinical trials. In addition, the course will address the calculation and interpretation of measures of disease frequency and association; the assessment of association versus causation in the interpretation of study results; and an introduction to issues related to the evaluation of chance, bias, confounding, and effect modification. Lectures will be complemented by seminars devoted to case studies, exercises, or critiques of relevant examples of epidemiologic studies.
Logistic Regression for Medical Research
David Wypij (Harvard T.H. Chan School of Public Health)
The course introduces students to the practice and application of logistic regression modeling for binary outcomes. Students will estimate, evaluate, and interpret binary data models arising from epidemiological studies, clinical trials, or other application areas. Topics include assessment of confounding and effect modification, use of indicator variables, model building methods, goodness-of-fit assessment, presentation of logistic regression models for reports and publications, and an introduction to conditional and ordinal logistic regression. Data sets from the medical and public health literature will be used as case studies to be analyzed using the Stata statistical program.
Joint Modelling of Longitudinal and Survival Data
Michael Crowther (Red Door Analytics)
The joint modelling of longitudinal and survival data has been an area of growing interest in recent years, with the benefits of the approach becoming recognised in ever widening fields of study. The models can provide both an effective way of conducting an analysis of a survival endpoint (e.g. time to death), influenced by a time-varying covariate measured with error, or alternatively correct for non-random dropout in the analysis of a longitudinal outcome (e.g. a biomarker such as blood pressure). This week-long course will provide an introduction to joint modelling through real applications to both clinical trial data and electronic health records, using examples in cancer, liver cirrhosis and cardiovascular disease. We will study the methodological framework, underlying assumptions, estimation, model building and predictions. We will also consider current developments in the field, looking at some of the many extensions of the standard framework, such as the ability to model multiple biomarkers and competing risks. The course will consist of lectures, classroom exercises, and computing exercises making use of the stjm and merlin packages in Stata, written by the course lecturer.
Block 3
Statistical Methods for Population Based Cancer Survival Analysis
Paul Lambert (Cancer Registry of Norway and Karolinska Institutet)
Paul Dickman (Karolinska Institutet)
Mark Rutherford (University of Leicester)
Therese Andersson (Karolinska Institutet)
Elisavet (Betty) Syriopoulou (Karolinska Institutet)
The course will address the principles, methods, and application of statistical methods to studying the survival of cancer patients using data collected by population-based cancer registries. We cover central concepts, such as how to estimate and model relative/net survival. We will cover the use of flexible parametric survival models, cure models, loss in expectation of life, and estimation in the presence of competing risks. Comparison of different approaches (e.g., to estimating and modelling relative/net survival) will be a focus of the course and participants will get the opportunity to apply and contrast a range of methods to real data. A large amount of timewill be devoted to exercise sessions where the faculty members will be available to work with participants individually or in small groups. The exercise sessions will also provide an opportunity for participants to discuss their own research projects withthe faculty (and with each other). We encourage potential participants to read the detailed course description at http://cansurv.net/.
Block 4
Research Methods in Health: Biostatistics
Marco Bonetti (Bocconi University)
This course is designed to provide the student with an understanding of the foundations of biostatistics and of the various statistical techniques that have been developed to answer research questions in the health sciences. Students will be introduced to methods for the comparison of outcome between two groups (t-test and non parametric tests), as well as the extension to the comparison of outcome across several groups (ANOVA); methods for the study of association between two continuous variables (correlation and linear regression); the analysis of contingency tables; the study of survival (time-to-event) data. The afternoon sessions are devoted to discussion and learning to use Stata to implement materials covered in the morning lectures.
Longitudinal Data Analysis
Garrett Fitzmaurice (Harvard T.H. Chan School of Public Health)
This course focuses on methods for analyzing longitudinal and repeated measures data. The defining feature of longitudinal studies is that measurements of the same individuals are taken repeatedly through time, thereby allowing the direct study of change over time. This type of study design encompasses epidemiological follow-up studies as well as clinical trials. The course covers many well-established methods for the analysis of longitudinal data when the response variable is continuous. Methods for discrete response variables (e.g., repeated binary responses and counts) are introduced, but not emphasized. An introductory course in biostatistics and a good background in linear regression analysis are prerequisites for this course.
Design of Registry-Based Studies and Randomized Clinical Trials
Gianluigi Savarese (Karolinska Institutet)
By the end of this course, participants will have a comprehensive understanding of how to structure and implement various types of registries. They will be equipped with the skills to design registry-based studies, effectively handling confounding factors. Additionally, attendees will learn to design and conduct clinical trials, analyze and interpret trial data, and address key statistical issues such as adjustments for confounders, biases, and performing subgroup analyses. These skills will be reinforced through practical examples, particularly from the cardiovascular field.
Block 5
Research Methods in Health: Epidemiology
Murray Mittleman (Harvard T.H. Chan School of Public Health)
This course will explore in greater depth the fundamental epidemiologic concepts introduced in Principles of Epidemiology (Week 1). The course will be taught with an emphasis on causal inference in epidemiologic researchwith afocus on chronic disease epidemiology and an emphasis on practical study design. Students will revisit the issues of confounding, selection bias, effect measure modification on the additive and multiplicative scales, and generalizability. Workshops will augment lectures to illustrate practical examples in the epidemiologic literature. The material covered in Principles of Epidemiology will be assumed of the students entering this course.
Survival Data Analysis
Sandra Eloranta (Karolinska Institutet)
This course introduces statistical methods for survival analysis with emphasis on the application of such methods to the analysis of epidemiological/public health cohort studies. Topics covered include methods for estimating survival probabilities and comparing survival between patient subgroups (the Kaplan-Meier method, log-rank test), and modelling survival (primarily Poisson regression and Cox proportional hazards regression). The course will emphasize both basic concepts of statistical modelling, such as controlling for confounding and assessing effect modification but also more complex topics including spline-based covariate adjustment and some approaches to address competing risks. Emphasis throughout the course will be on the concept of 'time' as a potential confounder or effect modifier (i.e. non-proportional hazards) and approaches to defining 'time' (e.g., time since entry, attained age, calendar time). In general, focus will be on interpretation and practical relevance of survival analysis rather than mathematical details. Guided, hands-on computer exercises that use the Stata software will be provided to reinforce the concepts and learn how to use and interpret the presented statistical methods.
Mediation and Interaction Analysis
Andrea Bellavia (Harvard T.H. Chan School of Public Health)
The course will introduce traditional and novel approaches for mediation and interaction analysis in clinical and epidemiologic research. Mediation analysis allows assessing social and biological pathways through which causal effects operate. Interaction analysis is instead critical to identify population heterogeneity in health outcomes risk. By investigating the mechanisms and the heterogeneity of causal effects, mediation and interaction analysis sit at the core of modern public health methodologies for precision medicine and causal inference. Fundamentals of the analytical approaches will be presented for dichotomous, continuous, and time-to-event outcomes, and discussion will be given as to when the standard approaches to mediation and interaction analysis are or are not valid. The relationship between traditional methods in the biomedical and social sciences will be discussed together with recent developments in causal mediation and interaction analysis. The course will also introduce some of the most recent developments in the field, including extensions to evaluate complex datasets with multiple mediators, high-dimensional data, and non-modifiable social constructs. The course will introduce Stata macros and commands to implement these approaches and will illustrate several applications from epidemiology and the social sciences. Basic knowledge of linear and logistic regression is recommended.
Sunday courses 1
Basics of Stata
Robert Thiesmeier (Karolinska Institutet)
This course is designed to introduce students to the basics of Stata. It will focus on the minimum set of commands everyone should know to organize their own work. Specific topics include data-management, data-reporting, graphics and basic use of do-files. By the end of this one-day course, the student should be capable of using Stata independently.
Data Visualization with Stata
Giovanni Capelli (University of Cassino and Southern Lazio)
The course introduces students to the logic and the strategies for visualizing data in Stata. Among the topics, the course will explore the issues in the choice of the most appropriate graphic (distributional, compositional or correlational) for different data and aims, and tips and tricks to prepare data for different graphical schemes. In particular, the power and flexibility of multiple "layers" in twoway Stata panels will be exploited. By the end of this one-day course, students will be able to produce Stata Graphs, and export them to JPG, TIFF or PDF formats for further applications.
Meta-analysis with Stata
Roberto D'Amico (University of Modena and Reggio Emilia)
Covers Stata commands for a variety of tasks regarding the combination of results from randomised controlled trials that consider binary, continuous and time to event outcomes: data preparation and input, fixed and random-effect models, forest plots, heterogeneity across studies, publications bias, sensitivity analysis, and meta-regression models.
Simulation studies with Stata
Nicola Orsini (Karolinska Institutet)
Monte-Carlo
simulations are a powerful tool to design and evaluate experimental and observational studies. While primarily being used to evaluate statistical methods, their application can be effective in learning how to design and calibrate a scientific investigations.
By using simulations to design a study, we can understand how to generate random data and mechanisms underlying it, such as random allocation, confounding, interaction, or missingness. Using computer experiments, we can compare the performance of alternative
analytical strategies, evaluate the ability of a complex study to point the investigator towards correct conclusions, and reason around sampling and systematic sources of variability in the design of a study. During this one-day course, students are given
an introduction to performing computer experiments using the Monte-Carlo method in Stata. Students learn how to simulate experimental and observational studies and generate data underlying common mechanisms (i.e., interaction, confounding, missing), while
controlling study characteristics (e.g., sample size, events) to maximise statistical power and quantify the frequency of inferential errors.
Sunday courses 2
Basics of Stata
Robert Thiesmeier (Karolinska Institutet)
This course is designed to introduce students to the basics of Stata. It will focus on the minimum set of commands everyone should know to organize their own work. Specific topics include data-management, data-reporting, graphics and basic use of do-files. By the end of this one-day course, the student should be capable of using Stata independently.
Multiple Imputation to handle missing data
Tim Morris (University College London)
This course will describe the problems caused by missing data and outline a principled approach to analysis. The emphasis will be on multiple imputation as a general purpose and flexible tool for handling missing data. Participants will learn about ‘full conditional specification’ to impute multiple variables with missing values, and learn to use it in Stata. The course will end with discussion of reporting analyses that use multiple imputation and understanding pitfalls.
Applied Machine Learning with Python
Andrea Giussani (Bocconi University)
This course is designed to introduce participants to the fundamentals of machine learning (ML)
and its growing impact on healthcare. In this one-day intensive session, we will explore the
essential concepts of ML, focusing on how it can revolutionize medical research and clinical
applications.
Starting with an introduction to ML, the course will cover why data generation and processing
are critical for effective model development. We will discuss techniques like cross-validation and
hyperparameter tuning, which ensure the accuracy and generalization of models. By addressing
common challenges, such as data bias and improper processing, participants will understand the
importance of clean, well-structured datasets in producing reliable outcomes.
As ML continues to evolve, we will dive into more advanced concepts like encoder-decoder
architectures, which have surpassed traditional ML models in processing medical images and
text data. These final sections will emphasize the differences between business-focused and
research-driven ML applications, providing a comprehensive view of how ML is shaping both
fields.
Participants will leave with a foundational understanding of how to apply ML techniques to
medical data, setting the stage for further exploration and specialization.
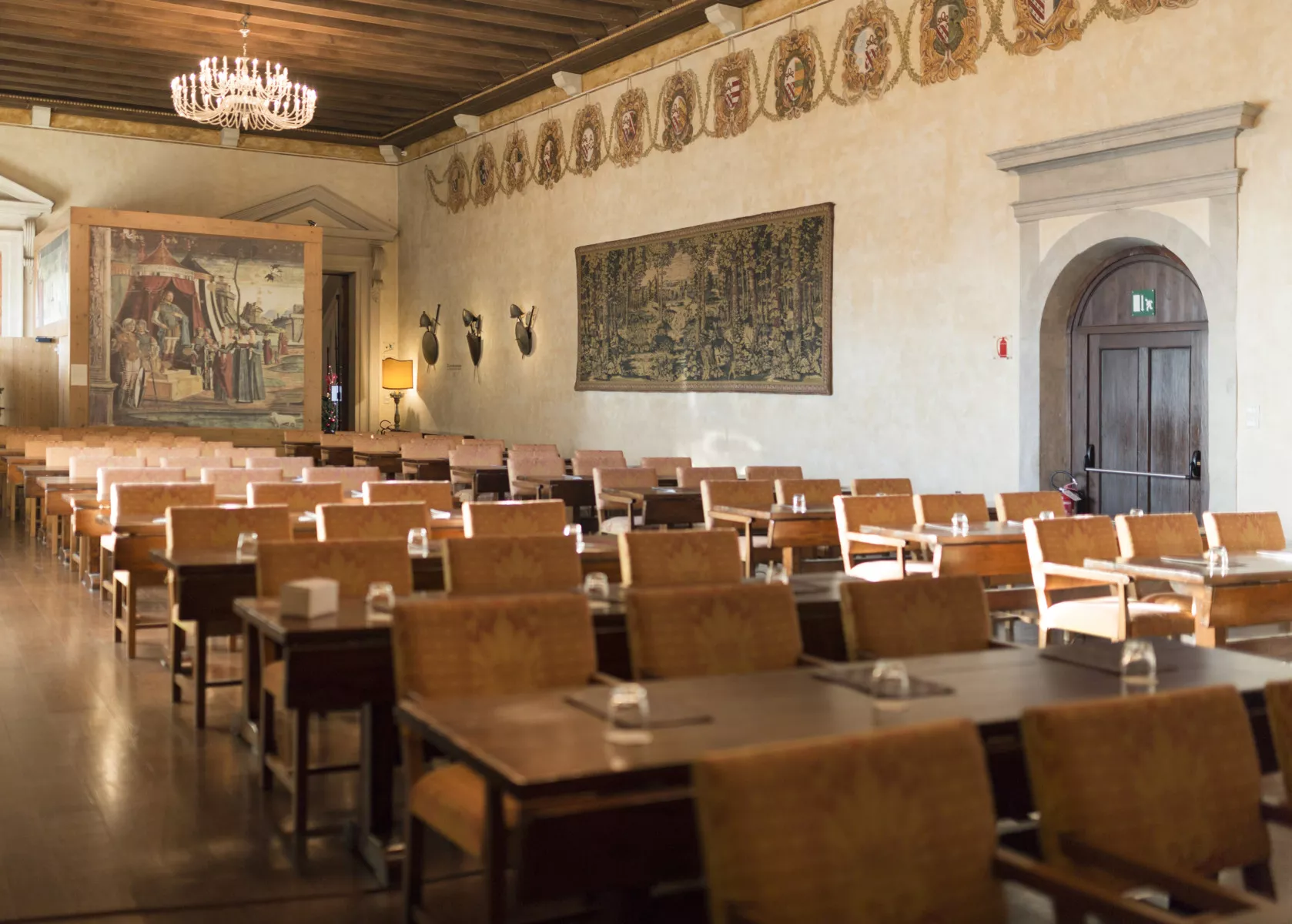